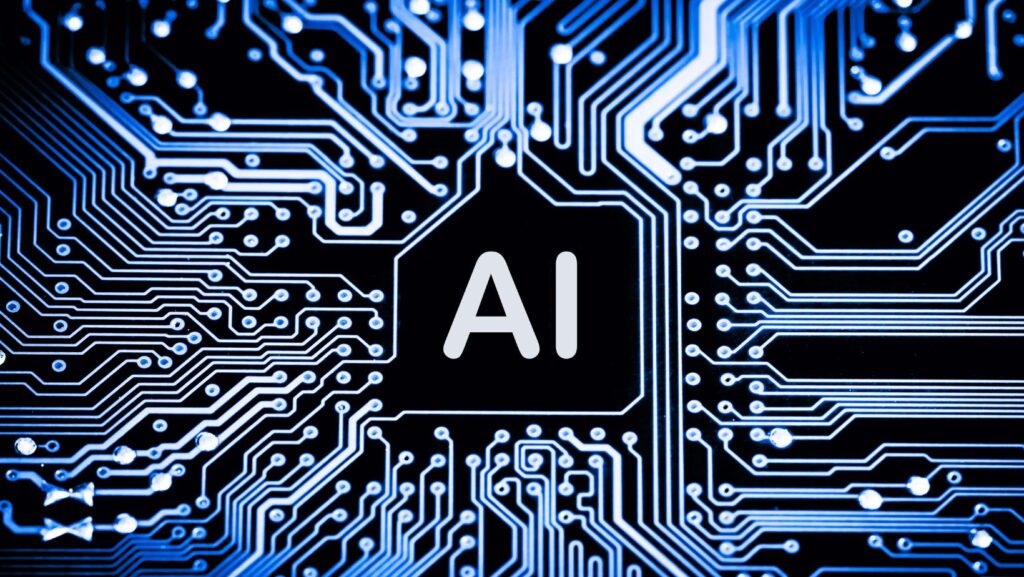
In the rapidly evolving landscape of artificial intelligence, Retrieval-Augmented Generation (RAG) stands out as a pioneering technology, offering significant advancements over traditional AI models. By integrating retrieval mechanisms with generative capabilities, RAG AI addresses the limitations of conventional systems, providing more accurate and contextually relevant responses.This article delves into the intricacies of RAG technology, explores its applications across various industries, and considers the future of this innovative approach. For a deeper understanding of the technical components, you can also explore K2view RAG IA.
Understanding Retrieval-Augmented Generation (RAG)
Definition of RAG Technology
Retrieval-Augmented Generation (RAG) is a cutting-edge AI model that combines retrieval-based methods with generative processes to enhance the quality and relevance of generated content. Unlike traditional AI models that rely solely on pre-trained data, RAG leverages external knowledge sources, allowing it to access real-time information and generate more contextually accurate responses.
Core Mechanisms of RAG AI
RAG operates through a dual mechanism: retrieval and generation. The retrieval component searches for relevant information from a vast repository of external data, while the generative aspect synthesizes this information to produce coherent and accurate outputs. This synergy between retrieval and generation enables RAG to provide more comprehensive and precise responses compared to traditional models.
Differentiating RAG from Traditional AI Models
Traditional AI models often face limitations in terms of data reliance and contextual comprehension. RAG, by integrating external knowledge retrieval, mitigates these issues, offering enhanced accuracy and real-time data access. This approach significantly reduces the risk of AI hallucinations, where models generate incorrect or nonsensical information based on insufficient data.
Technical Architecture of RAG
- Retrieval Mechanism Explained: The retrieval mechanism in RAG involves querying large databases to find relevant information, which is then used to augment the generative process. This ensures that responses are grounded in factual and up-to-date information.
- Generation Process and Knowledge Integration: The generation process in RAG synthesizes the retrieved data to create responses that are not only accurate but also contextually appropriate. This integration of external knowledge helps refine the output, making it more reliable and relevant.
- How RAG Enhances AI Response Accuracy: By tapping into external sources, RAG minimizes the reliance on static data sets, thereby increasing the accuracy and relevance of its outputs. This capability is particularly beneficial in dynamic fields where real-time information is crucial.
Advantages Over Traditional AI Models
- Reduced Hallucination Risks: RAG’s ability to access and utilize external data repositories ensures that the information it generates is based on accurate and up-to-date data, reducing the occurrence of hallucinations.
- Real-Time Information Access: The retrieval mechanism allows RAG to fetch the latest information, making it highly effective in environments where real-time data is essential.
- Improved Contextual Understanding: By integrating external knowledge, RAG provides a deeper contextual understanding, resulting in responses that are not only accurate but also highly relevant to the user’s query.
RAG Applications Across Industries
The versatility of RAG technology allows it to be applied across various industries, transforming how information is accessed and utilized.
Diverse Sector Implementation
RAG AI’s capability to enhance information retrieval and generation makes it suitable for a wide range of sectors, from healthcare to finance and beyond. Its transformative potential lies in its ability to solve complex information challenges and improve decision-making processes.
Healthcare and Medical Research
- Medical Literature Synthesis: In the healthcare sector, RAG can rapidly process and synthesize vast amounts of medical literature, providing practitioners and researchers with concise and relevant insights.
- Clinical Decision Support Systems: By integrating external medical databases, RAG enhances the accuracy of clinical decision support systems, offering physicians data-driven recommendations.
- Personalized Treatment Recommendation: RAG’s ability to access and analyze patient data and medical records enables it to provide personalized treatment recommendations, improving patient outcomes.
Financial Services and Analysis
- Risk Assessment Models: In finance, RAG aids in developing advanced risk assessment models by integrating real-time market data, enhancing the precision of financial predictions.
- Investment Research Automation: By automating the retrieval and analysis of financial reports and market trends, RAG streamlines investment research processes.
- Regulatory Compliance Support: RAG assists compliance teams by retrieving and analyzing regulatory documents, ensuring adherence to financial regulations.
Future of RAG and Emerging Innovations
Ongoing Technological Advancements
RAG technology is continuously evolving, with ongoing research focused on enhancing its capabilities and expanding its applications across various domains.
Potential Breakthrough Applications
As RAG technology advances, it holds the potential to revolutionize sectors by offering breakthrough applications that drastically improve the efficiency and accuracy of information processing.
Ethical Considerations and Challenges
Despite its benefits, RAG poses ethical challenges, particularly in terms of data privacy and the potential for misuse.
Addressing these concerns is crucial to ensuring the responsible deployment of RAG technology.
Enterprise AI Integration
- Custom Knowledge Base Development: Enterprises can leverage RAG to develop custom knowledge bases that are tailored to their specific needs, enhancing organizational intelligence.
- Intelligent Document Processing: RAG’s ability to process and analyze large volumes of documents streamlines workflows, improving operational efficiency.
- Enhanced Organizational Knowledge Management: By facilitating better information retrieval and management, RAG enhances organizational knowledge, leading to informed decision-making and strategic planning.
Emerging Research Directions
- Multi-Modal RAG Systems: Future research is exploring multi-modal RAG systems that integrate various types of data, such as text, images, and audio, to provide richer and more comprehensive outputs.
- Enhanced Contextual Understanding: Advancements aim to further improve RAG’s contextual understanding, resulting in even more accurate and relevant responses.
- Cross-Domain Knowledge Integration: RAG’s future applications may include cross-domain knowledge integration, allowing it to draw insights from diverse fields to solve complex problems.
Retrieval-Augmented Generation represents a significant leap forward in AI technology, offering transformative potential across industries. As RAG continues to evolve, its applications will undoubtedly expand, driving innovation and improving the efficiency and accuracy of information processing across sectors.